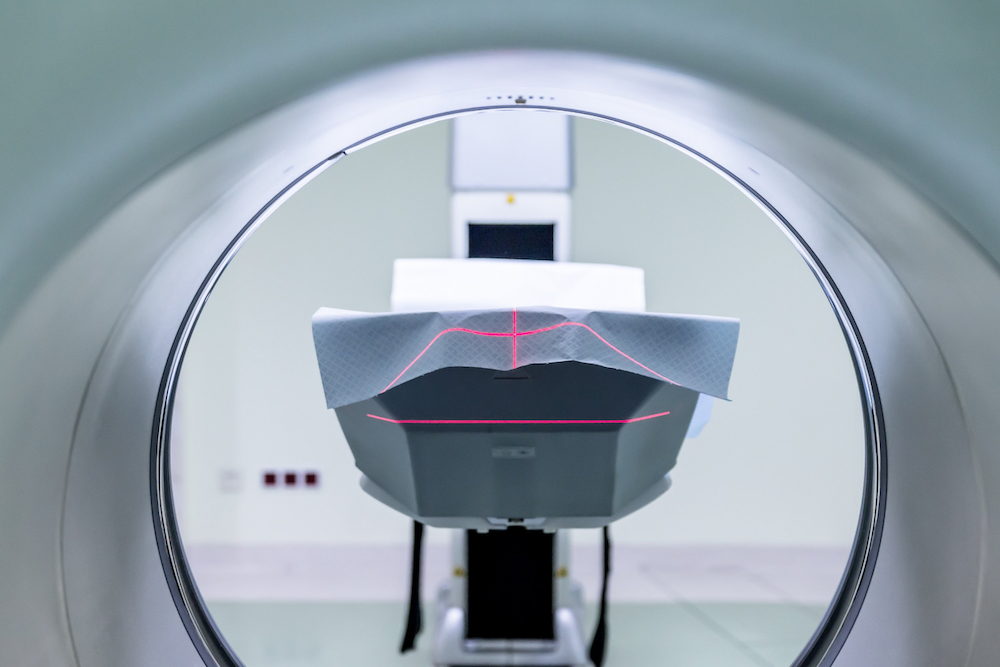
In the Age of AI Series, I dissect how companies in various industries are already using ML at the cutting-edge (for tangible business value, not research projects).
In this email, you’ll again learn about 3 cool companies:
- Using AI to improve radiation treatments for cancer: it’s one of the very few clinical-grade applications of deep learning in the medical industry. (Mahmudul Hasan, CEO of MVision AI)
- How AI is transforming the economics of the video game industry: which is important because video games are bigger than movies, sports, music, and all other entertainment markets combined. (Christoffer Holmgård, CEO of Modl AI)
- Making modern knowledge workers more productive using AI: an interesting upgrade to traditional project management software. (Dennis Kayser, CEO of Forecast)
1-Minute Summaries (if you’re short on time!)
1. AI for radiotherapy
The context:
Radiotherapy is a type of cancer treatment where you burn tumor cells with very precise rays.
The majority of cancer patients get prescribed radiotherapy — which amounts to 8-10 million patients per year. It’s the classic form of “precision medicine,” which has become a buzzword recently in the medical-tech field.
The problem:
Our story here begins after a patient has been prescribed radiotherapy. The next step is to create a proper treatment plan where the doctors have to exactly determine the tissue area that will be targeted, as well as the right dosage of radiation that won’t harm healthy cells.
Traditionally, this is done by doctors looking at 2D scans with their naked eye, and scribbling some notes. Not only is this a very manual process, and requires a LOT of training and experience, there’s a lot of inconsistency — the same doctor will annotate the same scan differently each time.
This creates operational inefficiencies due to which the treatment plan sometimes takes up to 2 weeks to get prepared.
The solution:
You can use deep learning to automatically segment the cancerous tissue from healthy cells, and streamline the treatment planning workflow.
My guest was Mahmudul Hasan, whose company MVision AI builds software for that. They’re already in 20 countries, and he claims that certain hospitals have even been able to conduct same-day treatments.
In the full post, we go in-depth into how you build a clinical-grade deep learning product, and design an engineering process for it.
2. AI for video games:
The context:
Video games are an extremely competitive industry, because the payoffs are so huge.
At the same time, most video games fail, and most games are free to play — making money through in-app purchases and ads.
So game dev is even worse than being a venture capitalist:
- First you need to make many games per year, as cheaply as possible just to have one hit.
- After it’s a hit, you have to spend a lot more resources on retention and engagement — constantly releasing new levels and features, etc to keep players interested and keep monetizing.
The problem:
A lot of game development is notoriously hard, boring, manual work, especially testing/QA.
If we can automate parts of that process, it can be the difference between life and death for some game studios.
The solution:
You can’t do this for every game, but you can use AI for:
- Automated testing: Bots that intelligently explore the game and find the most important glitches.
- Engagement: Bots that can play impressively well against human players, by learning over time.
- Retention: Automatically generating new levels
My guest was Christoffer Holmgård, CEO of Modl AI, which works on all 3.
In the full post, we go deeper into the video games landscape and the systems Modl AI is building.
3. AI for project management
The core idea around upgrading PM software is that it’s too rule-based, and ignores a lot of context:
- The average knowledge worker works on 3.6 projects at a time, not just one.
- The actual work done in each company is unique, but the nature of work is usually commonplace. (For example: Many journalists write 500-word articles, though the actual articles are different. Many developers write front-end code, though the actual code is different.)
The problem:
Most PMs and traditional software are incapable of using these facts while project planning.
It leads to poor predictions of “how long it will take,” burnout, missed deadlines, and the wrong people working on the wrong things at the wrong time.
The solution:
Turns out, you can use ML to do some things that only good human PMs would do:
- Forecast how long it takes to complete certain types of work, just by reading the task descriptions
- Learn the individual working capacities of employees, to help use their effort more effectively
My guest was Dennis Kayser (who is obviously related to Kaiser Wilhelm II but denies it out of modesty), CEO of Forecast.
I was quite skeptical about how well ML can work for project management. So in the full post, we discuss in-depth how they do it!
Want this newsletter in your inbox? Sign up here!
P.S. Ethics Policy: I don’t own stock in guests’ companies, nor do I get paid by them in any form for any reason at the time of publishing, unless specifically stated. Episodes are also not intended to be an automatic endorsement of any company or its products and services. These opinions are 100% my own as an independent observer and educator.