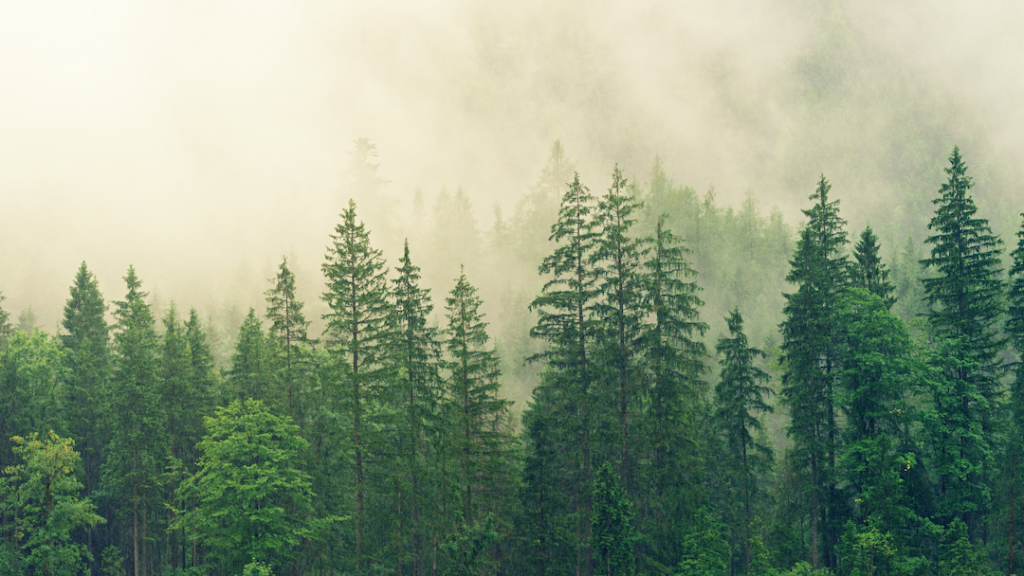
This is a new edition of my Age of AI Series, where I dissect how companies in various industries are already using machine learning (for tangible business value, not research projects). Want to get this newsletter? Sign up here!
In this email you’ll learn about 3 companies:
- Using ML to conserve forests — for forest management and conservation: CollectiveCrunch
- Using ML to help print newspapers go be more like Netflix: iMatrics
- Building equipment and appliances that use ML to get better on their own: Ekkono
First, a 2-minute summary if you’re short on time:
AI in Forestry
- The forestry business at its heart is about selling wood. This means, their core “merchandise” is lumber or wood pulp.
- Since forests are huge, non-uniform and constantly changing (species, density, heights of trees, changing seasons, etc), the process of taking an accurate “inventory” of their merchandise is an expensive challenge.
- Conventional methods of taking this inventory supposedly have error rates of as much as 20-40%. It really affects the bottom line of forestries.
- Turns out, by using ML you can combine many sources of data (satellite imagery, LiDAR scans, manual sampling, etc) to reduce the error rate, creating real business value.
- I spoke with Rolf Schmitz, CEO of CollectiveCrunch which does exactly that. We get into the weeds (pun intended) of forestry and carbon economics, and other stuff: (Link)
AI for “Newspapers”
- News media has been struggling: offline, print subscriptions are dropping anyway; online, ad revenue is dropping too and people find paywalls annoying.
- The ideal scenario for news media is to have customers pay for online subscriptions, and retain them — a problem that Netflix and streaming companies have already been trying to solve for a decade.
- One of the key aspects of that is a personalized recommendation engine, which most news orgs don’t have.
- To build such an engine, it’s critical to tag and categorize news articles properly, which is not only time-consuming for journalists, but also VERY hard to do properly.
- I met Mari Ahlquist, CEO of iMatrics which uses ML to do the tagging. This interview is slightly more technical. (Link)
AI for Self-Learning Devices:
- Edge ML is about devices collecting their own data and learning from it themselves, without having to send data to the cloud or back-end for processing.
- In industry right now, applications fall under two umbrellas:
- One is predictive maintenance — instead of getting routine check-in visits, the machine can learn to sense the need for maintenance on its own, and caution about impending damage.
- The other is personalized products — devices learning about users’ preferences over time, eg: a robotic lawn mower learning about YOUR lawn and its eccentricities, giving you a better customer experience. This would also tempt you to buy other lawn care devices from the same company, so that the learnings could be transferred.
- But building edge ML and connected devices is challenging, because the infrastructure and suite of tools available to developers is still maturing.
- I met Jon Linden, CEO of Ekkono which builds tools for edge ML developers. We cut the buzzword-bingo that’s common in this industry, and get down to the actual facts. (Link)
Key takeaways (5 minute read)
ML in forest industry (full post)
From an ML standpoint, getting high-quality data is the big headache, and then there’s the question of how you deliver the predictions and other outputs in an interactive, flexible, user-friendly interface.
From a business standpoint, Rolf’s team made some key decisions in the beginning — they only do data analytics, and let the customer fly their own drones to collect raw data. They didn’t want to be an all-in-one services company that sends people to travel around jungles around the world and gather data on behalf of customers.
Having a SaaS model makes the company much more scalable, but Rolf confesses that they could have had faster growth and expansion into more markets if they were a “full stack” solution that included data collection services. At the same time, doing that would open a whole new can of worms that a lean company can’t handle without excessive cash burn, so I’m inclined to think that this was the right decision.
I’m also curious about how much the perceived value of their product in the eyes of customers (or company in the eyes of investors) is directly influenced by the commodity price trends of lumber, which is a natural resource. Rolf and I didn’t have enough time to discuss how this affects the way they define their market and product roadmap over the long term.
Lastly, I also found it interesting that sustainability is quickly becoming a real business focus in forestry, due to the growing market for carbon credits. Planting trees is the cheapest form of carbon capture, and forestry companies are using this to create a new source of revenue. But doing this profitably still requires having accurate inventory of the forest, which brings us back to companies like Collective Crunch — and if this trend continues, I’m optimistic that Rolf and his team are only at the beginning of a long successful journey with the technology they’ve built!
News tagging using ML (full post)
Tagging in general is a problem for which it’s easy to build a bad solution, and very hard to build a good solution. Fundamentally, it’s pretty trivial (you could build a simple automated tagging software as a college project), but doing it properly for something as sensitive as news is tricky and sophisticated. Examples:
- A lot of news is contextual — an article about a famous golf player’s divorce may not even mention the word “golf,” but would ideally need to be tagged as such. Mari said that to do this, they connect to datastores like Wikipedia to add extra layers of information for the system to consider while tagging.
- The world is changing and the news is always, well, new. How do the tags evolve to keep up? (This came up during the coronavirus pandemic as a whole new category was suddenly born.) The answer is that you can’t predict the future, so you just have to do that manually. Making the system learn over time isn’t straightforward.
- If the tags decide the recommendations and the order in which news appears on organization’s website and feeds, there’s a chance that journalists will deliberately write articles to always include the hottest tags. Mari said they had to take a lot of precautions while creating the system to ensure that doesn’t happen, but it remains a fundamental problem.
My personal opinion is that their solution almost sounds too strong to be true! Based on the maturity of the product Mari described, I was skeptical that they could achieve it in just a few years with a fairly small team of engineers, given the amount of R&D and iteration involved. Most typical “start-ups” either give up and pivot towards other problems, or have a huge headcount by the time they get to that level of product maturity. Mari said that their customers’ engineering teams often share the same disbelief.
But since the company has its roots in academia (it grew out of a university research project), I’m more inclined to believe that their product really is already as complicated as Mari described. Moreover, the technology they’ve developed is very transferable to many other types of unstructured content, not just limited to news media, so I am sure they have even better prospects ahead of them.
Building connected devices with edge ML (full post):
The feasibility of such products heavily depends on the hardware sensors available on the device, which I see as the core constraint for this tech. Everything else — the software tools for ML, connectivity tools, semiconductor chips for the embedded computers, etc are getting better.
Ekkono provides a software toolkit for doing edge ML. To buy Ekkono, a customer already needs to have invested in an in-house team working on connected devices, so it’s still a market of early adopters. Some customers ask Ekkono to do everything for them, but being a product-focused company that doesn’t want to carry a services business model, they partner with “solution providers” or consultants to provide that instead.
Jon agrees that while offering end-to-end services would have helped sign more deals, it would have thrown off the company’s focus. (We’ve seen this theme before in Episode 2, where Rolf’s company CollectiveCrunch, also a pioneering company in an early-adopter market, made a similar strategic call not to be a services company.)
As an industry observer, Jon’s take on IoT is that while the landscape is as “hot” as it’s ever been, it hasn’t matured as much as people expected, and he also admitted that he’s surprised their space isn’t more crowded.
That being said, the space is growing enough that they have plenty of paying customers, and being the first mover in a market like this is THE big advantage for a company like Ekkono. After all, if you want to be the go-to toolkit for building edge ML applications, you don’t wait for the market to “mature”; you enable it to happen.